Welcome to the Prognostics and Health Management Group
Dedicated to providing a research and knowledge base to support the advancement of diagnostics, prognostics, and system health management.
-
Prognostics is the process of monitoring the health of a product and predicting its remaining useful life (RUL) by assessing the extent of deviation or degradation from its expected state of health in its expected usage conditions.
-
Health Management utilizes prognostic information to make decisions related to safety, condition-based maintenance, ensuring adequate inventory, and product life extension.
-
Prognostics and Health Management (PHM) permits the evaluation of a system’s reliability in its actual life-cycle conditions.
The Prognostics and Health Management (PHM) Group has a multi-faceted approach to PHM focused on demonstrating that health monitoring can be implemented using a variety of methodologies, tools, and analyzing techniques for effective prognostics. Our approaches for PHM implementation include: (1) the use of expendable devices, such as canaries and fuses that fail earlier than the host product to provide advance warning of failure; (2) monitoring and reasoning of parameters that are precursors to impending failure, such as shifts in performance parameters; and (3) modeling of stress and damage in electronic parts and structures utilizing exposure conditions (e.g., usage, temperature, vibration, radiation) to compute accumulated damage.
The PHM Group conducts research and development of prognostics and health management applications for electronic products and systems, as well as systems-of-systems. The research focuses on computational algorithms, advanced sensors and data collection techniques, condition-based maintenance, PHM for the application of in-situ diagnostics and prognostics. The group is using physics-based models along with empirical models for prognostics. It is pioneering the use of a fusion approach, which combines physics of failure and data-driven methods for accurate prognostics and diagnostics.
The goal of the group is to develop novel ways to identify anomalies and patterns within very large data sets containing multiple parameters both qualitative and quantitative and has developed real-time reduced-order modeling for failure prediction. Work in the areas of reliability modeling and prediction, pattern recognition, time series forecasting, machine learning, and fusion technologies is ongoing. The group is evaluating the use of intelligent reasoning technologies to model and manage the life cycle of electronic products. In addition, optimal maintenance planning and business case development to assess the return on investment associated with the application of PHM to systems is being researched by the group.
The PHM Group collaborates with industry and research partners to develop advanced sensors for diagnostics and prognostics applications. Applications such as tamper proof low-cost autonomous sensors that incorporate wireless communication, and high onboard memory capacity and can be attached to any product with minimal interference to the functioning of that product are being developed. The PHM Group enables real-time prognostics and health management of electronic products in their application environment.
PHM Application Areas
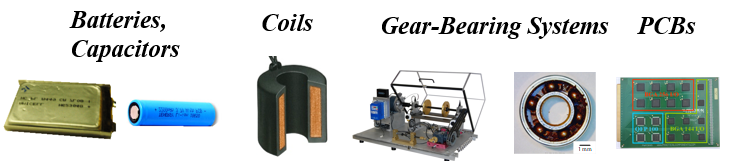
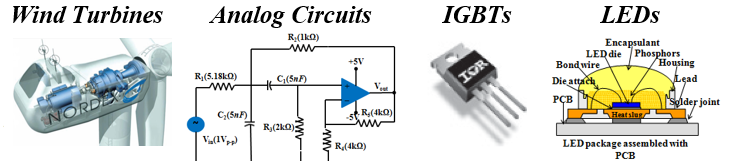
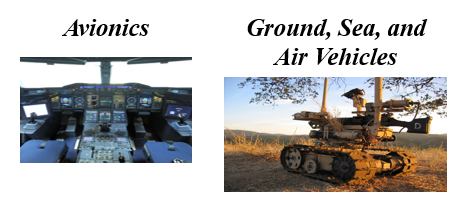
Recent Research Projects
Lockheed Martin: Remaining Useful Life Generation and Indicator Algorithms (2015-2016)
NSF: Prognostic Methods for Battery Management Systems (2012-2015)
US Navy: Condition Based Maintenance Plus (CBM+) Advanced Fault Diagnostics (2013-2014)
US Navy: Ground Tactical Value Prognostic Health Management for U.S. Marine Corps (2011)
US Army Research Laboratory: Autonomous Prognostics and Health Monitoring Systems for Weapons Platforms (2010)
US Army: Dynamic Data-Driven Prognostics and Condition Monitoring of On-board Electronics (2008 – 2010)
NASA: Reliable Diagnostics and Prognostics for Critical Avionics Systems (2008-2011)
Lockheed Martin: Prognostics of Systems-of-Systems (2007)
US Navy: Advanced Prognostic and Health Management (PHM) and Model Based Prognostic Useful Life Remaining Capabilities for Aircraft Tactical Information and Communication (2007)
Office of the Secretary of Defense: A Prototype Web-based Health (Prognostics) Assessment of a Test System (2006)
Dell: Baseline Characterization of Notebook Computers Subject to Changing Environmental and Operational Conditions for Diagnostics and Prognostics (2006)
NASA: Remaining Life Assessment of Space Shuttle Solid Rocket Booster Electronic Hardware (2004)
NASA: Remaining Life Assessment of Space Shuttle Remote Manipulator System (2001)
Insulated Gate Bipolar Transistors (IGBTs)
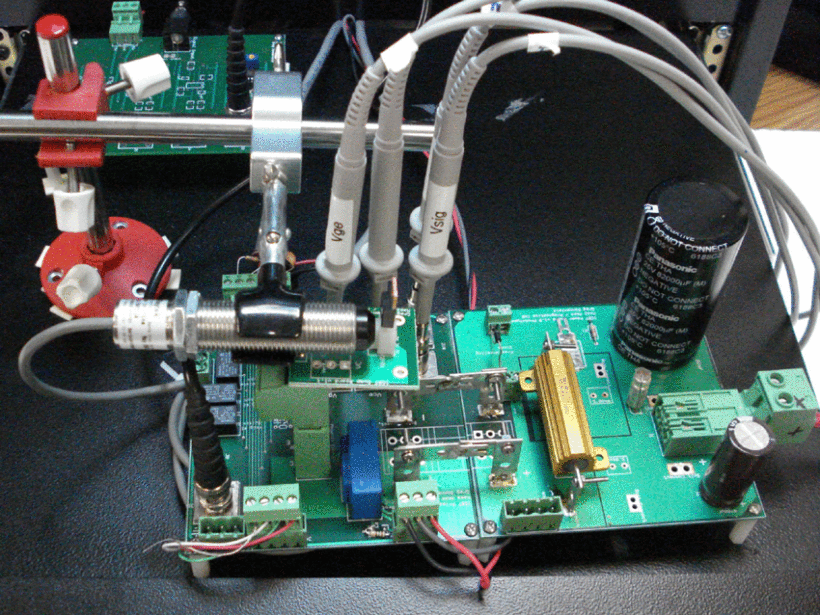
In power electronic system, insulated gate bipolar transistors (IGBT) are one of the most common semiconductors that switch current under high voltage. In order to build prognostic model for IGBT, precursor parameters have been identified. IGBT were subjected to thermal overstress tests using a transistor test board until device latch-up. During the test, current, voltages, and temperatures were monitored online at different locations. The aged parts were observed to have shifts in capacitance-voltage measurements as a result of trapped charge in the gate oxide. The collector-emitter ON voltage VCE(ON) showed a reduction with aging. The reduction in the VCE(ON) was found to be correlated to die attach degradation, as observed by scanning acoustic microscopy analysis. The collector-emitter voltage, and transistor turn-off time were observed to be precursor parameters to latch-up. Based on these precursors, a physics-based prognostic model was developed.
A Prognostic Approach for Non-Punch Through and Field Stop IGBTs, N. Patil, D. Das, and M. Pecht, Microelectronics Reliability 52 (2012) 482-488, 2012.
Precursor Parameter Identification for Insulated Gate Bipolar Transistor (IGBT) Prognostics, N. Patil, J. Celaya, D. Das, K. Goebel, and M. Pecht, IEEE Transactions on Reliability, Vol. 58, No. 2, pp. 271-276, June 2009.
Light Emitting Diode (LED)
Light-emitting diodes (LEDs) are widely used for general lighting and display applications. As the demand for LEDs has grown, the need to quickly qualify them has emerged. To address this issue, a prognostics-based qualification method was addressed. The method used an efficient relevance vector machine regression model that reduces the qualification testing time of LEDs from 6000 h (as recommended by industry standards) to 210 h. During the test, the remaining useful life of a LED was predicted by deriving the relationships between the spectral power distribution of a light and light output (lumen), and color coordinate values.
Batteries
- Model-based Satate of Charge Inference
Battery state of charge (SOC) estimation is a crucial function of battery management systems (BMSs), since accurate estimated SOC is critical to ensure the safety and reliability of electric vehicles. CALCE team evaluated widely used battery SOC online estimation methods. The methods were compared and evaluated in terms of tracking accuracy, convergence time, and robustness for online estimating battery SOC. Among tested methods, an incremental OCV test is recommended for predetermining the relationship between open circuit voltage and SOC for estimating battery SOC online in BMSs.
- Physics-based Satate of Charge Inference
Current commercial BMSs do not provide adequate information in real time to mitigate issues of battery cells such as thermal runway. CALCE team inserted fiber optic Bragg grating (FBG) sensors inside lithium-ion battery coin cells to monitor the condition of battery cells. Strain and internal and external temperatures were recorded using FBG sensors. The preliminary results present scanning electron microscope (SEM) images of electrode degradation upon sensor integration during cell charge/discharge cycles.
The FBG sensor was placed on the inside of the coin cell between the electrodes and the separator layers towards the most electrochemically active area. Results show stable strain behavior within the cell and about 10 °C difference between the inside of the coin cell and the ambient environment over time during charging/discharging cycles. By modeling this expansion suing a strain gauge, a prognostics model was developed.
The same phenomena can be also monitored using ultrasound sensors. The amplitude or time-to-flight of the ultrasound can be used to assess the degree of swelling expansion, delamination, voiding and electrode ruffling of the batteries. These measurement can be used in developing a metric to explain the degradation level of batteries.
Preliminary Study on Integration of Fiber Optic Bragg Grating Sensors in Li-Ion Batteries and In Situ Strain and Temperature Monitoring of Battery Cells, Aleksandra Fortier, Max Tsao, Nick D. Williard, Yinjiao Xing, and Michael Pecht,Energies, Vol. 10, Issue 7, pp. 1-11, June, 2017.
Electronic Circuits
Degradation of electronic components is typically accompanied by a deviation in their electrical parameters from their initial values, which can ultimately lead to parametric faults in electronic circuits. Existing approaches to predict parametric faults emphasize identifying monotonically deviating parameters and modeling their progression over time. However, in practical applications where the components are integrated into a complex electronic circuit assembly, product or system, it is generally not feasible to monitor component-level parameters. To address this problem, CALCE team developed circuit health estimation methods using machine learning algorithms. The methods exploit features that are extracted from responses of circuit-comprising components exhibiting parametric faults, instead of the component-level parameters.
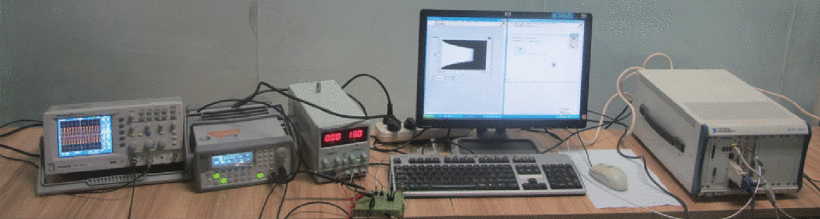
Electronic Circuit Diagnosis with No Data, Varun Khemani, Michael. H. Azarian, and Michael. G. Pecht, IEEE International Conference on Prognostics and Health Management, June 2019, DOI: 10.1109/ICPHM.2019.8819424
Electronic Circuit Health Estimation Through Kernel Learning, Arvind Vasan and Michael Pecht, IEEE Transactions on Industrial Electronics, Vol. PP, No. 99, July 2017.
Signal Model-Based Fault Coding for Diagnostics and Prognostics of Analog Electronic Circuits, Zhenbao Liu, Taimin Liu, Junwei Han, Shuhui Bu, Xiaojun Tang, and Michael G. Pecht , IEEE Industrial Electronics Society, Vol. 64, Issue. 1, PP. 605-614, 2017, DOI: https://doi.org/10.1016/j.microrel.2016.12.015 .
Coils
Electromagnetic induction coils are widely used in a variety of applications, many of them safety critical. The insulation around the wire in an electromagnetic coil accounts for a significant portion of the failures in solenoid-operated valves and in electric motors. CALCE team developed a method to detect the degradation of insulation used in low-voltage applications by assessing changes in impedance responses. The results indicate that coil impedance, resolved into resistance and reactance, evolves differently when the coil is subjected to different loading conditions, which reflects insulation degradation signatures due to different failure mechanisms. This method can be used to assess the insulation life of an electromagnetic coil, allowing replacement prior to the formation of harmful shorts or critical coil opens.
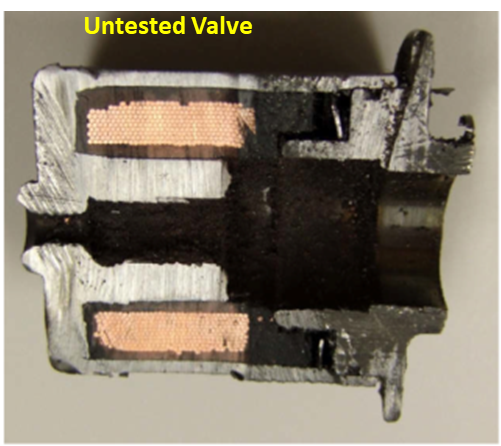
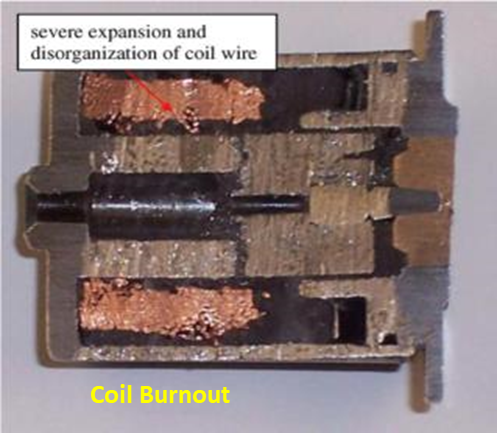
Impedance-Based Condition Monitoring for Insulation Systems Used in Low-Voltage Electromagnetic Coils, Noel Jordan Jameson, Michael H. Azarian, and Michael G. Pecht, IEEE Transactions on Industrial Electronics, Vol. 64, No. 5, pp. 3748-3757, May, 2017.
Bearings
Although bearings have been the focus of PHM for many years, a number of challenges remain. CALCE has focused on the development of methods to address: complexity of sensor information, high rates of false positives and negatives in anomaly detection, and inaccuracy of predicted remaining life. A wide range of characteristics are monitored and analyzed for fault detection, diagnosis of defects, and prognostics: vibration; acoustic emission; acoustic sound; wear debris; nano-hardness; surface topography; lubricant chemistry; motor current; rotational speed.
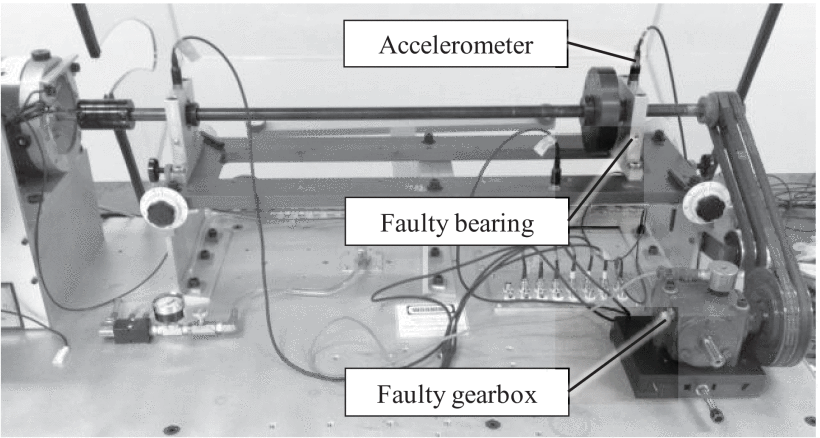
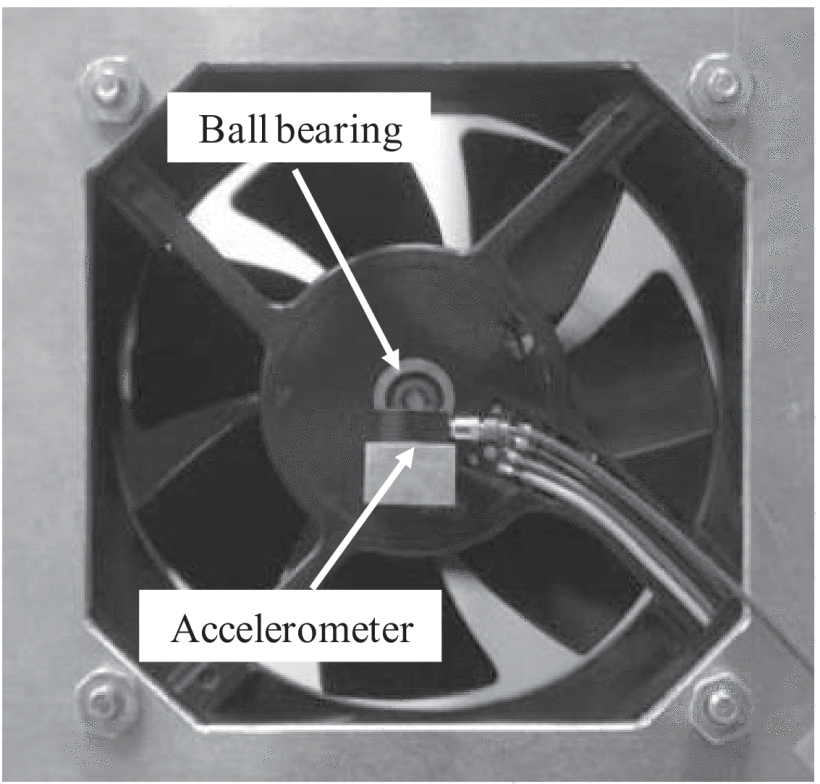
Motor bearing fault detection using spectral kurtosis-based feature extraction coupled with k-nearest neighbor distance analysis, Jing Tian, Carlos Morillo, Michael H. Azarian, Michael Pecht, IEEE Transactions on Industrial Electronics, Vol. 63, No. 3, 1793-1803, March 2016.
Health Monitoring of Cooling Fan Bearings Based on Wavelet Filter , Wei He, Qiang Miao, Michael Azarian, and Michael Pecht, Mechanical Systems and Signal Processing, Vols. 64-65, pp. 149-161, December, 2015, DOI: 10.1016/j.ymssp.2015.04.002.
Failure Mechanisms of Ball Bearings Under Lightly Loaded, Non-Accelerated Usage Conditions , Hyunseok Oh, Michael H. Azarian, Carlos Morillo, Michael Pecht, and Edward Rhem, Tribology International, Vol. 81, pp. 291-299, DOI: 10.1016/j.triboint.2014.09.014.
Solder Joint
Solder joints are among the most common failure sites in electronic assemblies. CALCE team developed a prognostic approach that allows for the remaining useful life prediction of solder joints using an RF impedance analysis and the Gaussian process (GP) regression. While the solder joints were exposed to a mechanical stress condition to generate fatigue failures, the RF impedance of the solder joint was continuously monitored. The RF impedance provided an early indication of the impending solder-joint failure in the form of a gradual increase prior to the end of life. A GP model was applied to the RF impedance obtained from the fatigue tests in order to estimate the remaining life of the solder joint in real time. It was demonstrated that the GP model successfully predicted the time to failure of the solder joint with high accuracy prior to failure. The prediction performance was also evaluated using prognostic metrics.
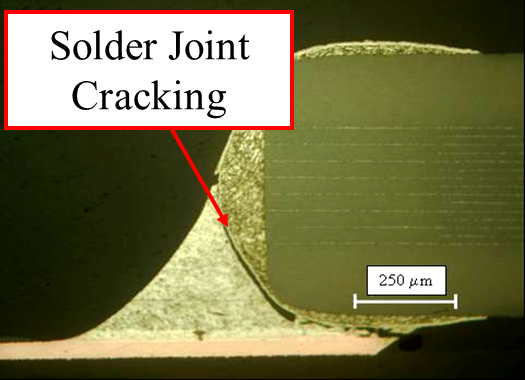
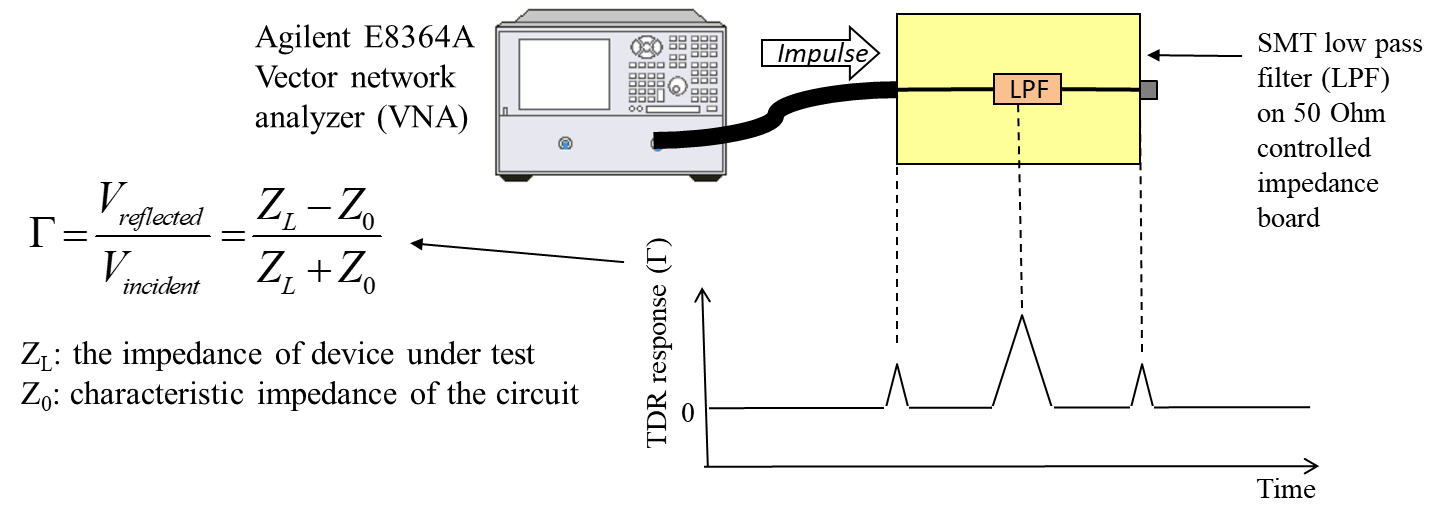
Remaining-Life Prediction of Solder Joints Using RF Impedance Analysis and Gaussian Process Regression , Daeil Kwon, Michael H. Azarian, and Michael G. Pecht, IEEE Transactions on Components, Packaging and Manufacturing Technology, Vol. 5, No. 11, November 2015.
Automotive Safety Components
Some component issues in automotive vehicles, such as wheel imbalance and wheel misalignment, can cause abnormal vibrations while driving. CALCE team developed diagnostics of six faulty conditions that generate abnormal vibrations. For each faulty condition, vibrations were collected using three-axis accelerometers. The vibrations were analyzed by decomposing frequencies using short time Fourier transformation. Different frequency signatures were found from different faulty conditions. To diagnose failures, a deep convolutional neural network was trained.
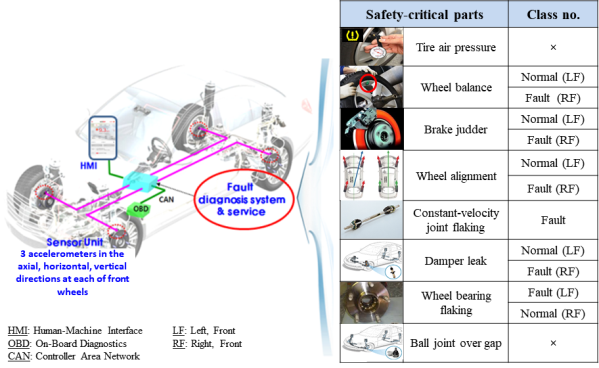
A Comparative Study of Deep Learning-Based Diagnostics for Automotive Safety Components Using a Raspberry Pi, Namkyoung Lee, Michael H. Azarian, Michael G. Pecht, Jinyong Kim, and Jongsoon Im, IEEE International Conference on Prognostics and Health Management, June 2019.
CALCE PHM Software
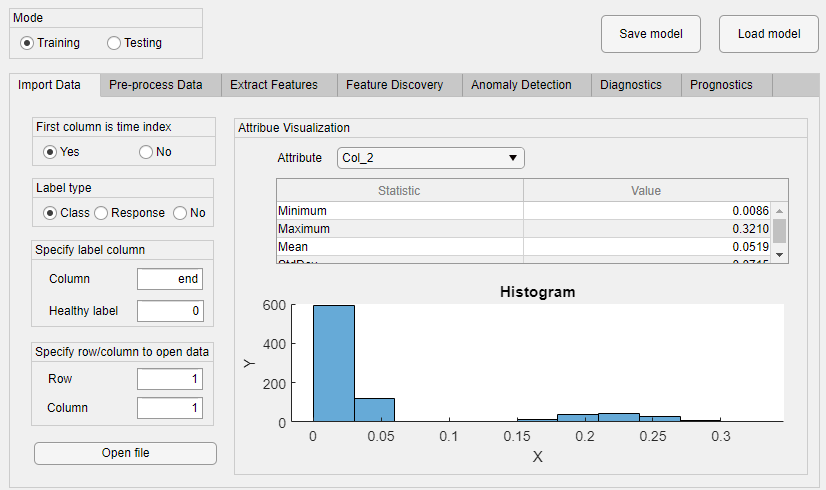
CALCE has developed a software that builds a prognostics and health management system without coding. The software uses graphic user interfaces that guides users to follow each process to build the system. For each process, CALCE incorporated machine learning algorithms that are effective to handle each process. Users can test multiple algorithms with few clicks and therefore, compare the performance of algorithms quickly. The full list of implemented machine learning algorithms is shown below:
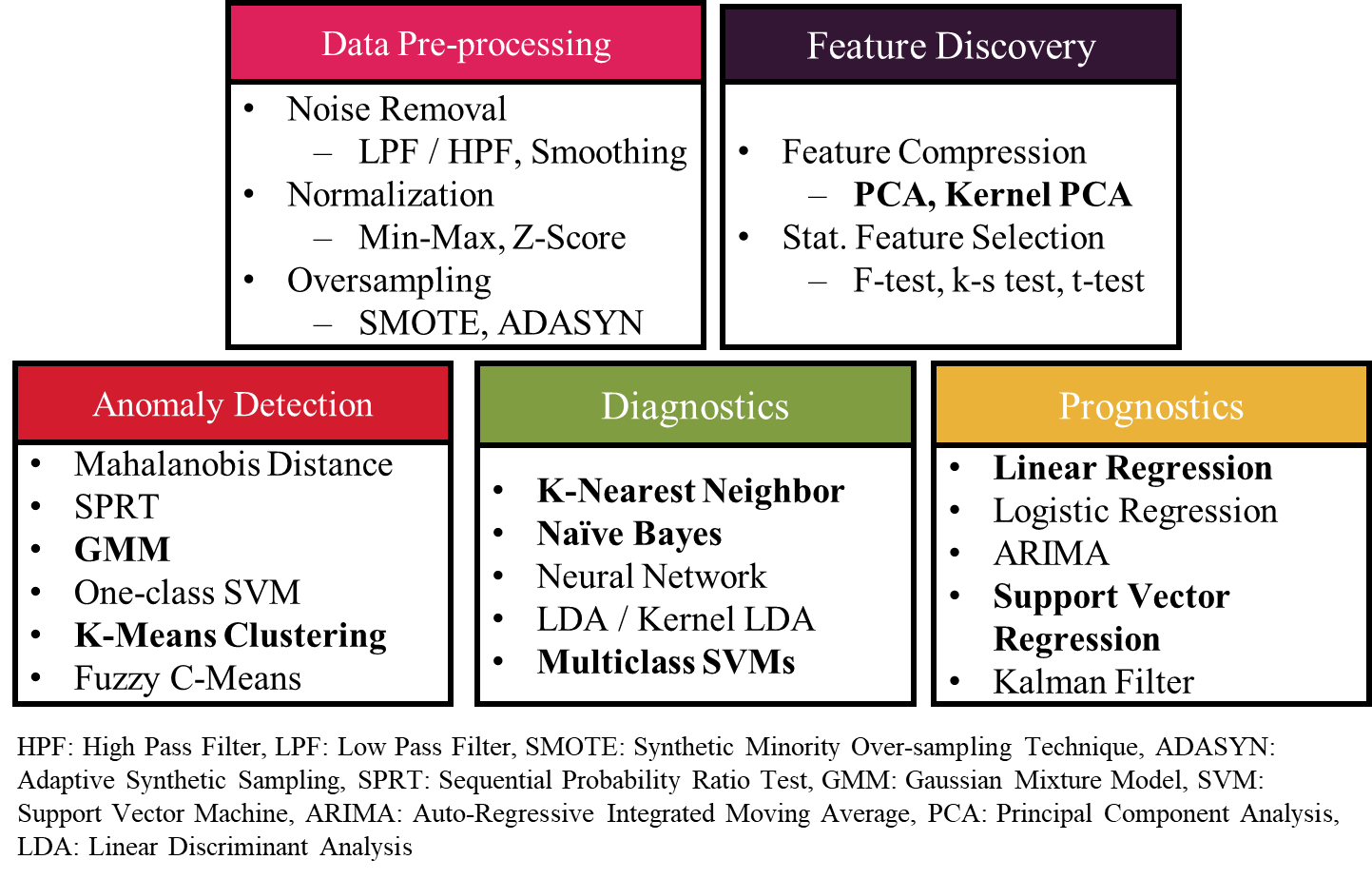
For more information contact pecht@calce.umd.edu, and mazarian@calce.umd.edu
CALCE has developed a stochastic decision model that determines when scheduled maintenance makes good business sense, i.e., makes possible a business objective such as a balance of cost and availability. The model enables the optimal interpretation of life consumption monitoring damage accumulation or health monitoring precursor data, and applies to failure events that appear to be random or appear to be clearly caused by defects.
The initial version of the tool focused on modeling and optimizing the cost avoidance associated with the application of PHM to systems. The tool implements a stochastic discrete event simulation applied to single and multi LRU systems where the LRUs can have no PHM structures, fixed interval maintenance, life consumption monitoring, or precursor to failure health monitoring. The tool can be used to optimize safety margins and prognostic distances for single LRUs and to determine best maintenance strategies for multiple LRU systems. In Spring 2007, the tool was extended to address false positives. The tool has been used to perform ROI studies on single LRU systems. The metrics computed by the tool include:
-
Life cycle cost (of a socket or group of sockets)
-
Failures avoided
-
Operational availability
Recently the tool was enhanced to model PHM implementation costs (non-recurring development costs, recurring costs at the LRU, socket and multi-socket levels, and infrastructure costs. A revenue model is also being added to provide to differentiate between unscheduled maintenance that occurs before during and after missions.
For more information contact sandborn@calce.umd.edu
