News Story
Access New Hybrid RUL Prediction of Li-ion Batteries Article Now
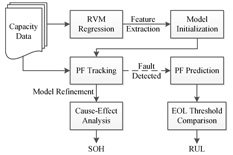
“Validation and verification of a hybrid method for remaining useful life prediction of lithium-ion batteries,” Journal of Cleaner Production, Professor Pecht, YongZhi Zhang, Rui Xiong, and HongWen He.
Abstract: Remaining useful life (RUL) prediction is an effective technique to provide the lifetime information of lithium-ion batteries for both manufacturers and users. The current methods for battery RUL prediction face two challenges—first, a large amount of training data is required for accurate RUL prognostics, and, second, the prediction performance of the prognostic algorithms for battery failure cannot be verified effectively. To reduce the training data, a fusion technique consisting of relevance vector machine and particle filter (PF) was developed to construct an aging model of the battery for RUL prediction. Based on the fusion technique, the training data can be reduced to 30% of the entire degradation data. Then, a validation and verification framework based on the Monte Carlo method was introduced as a baseline to calibrate the number of particles and model noise level of PF. The calibrated PF predicted the failure time 18 cycles earlier than the real value within a prediction horizon of 560 cycles.
Please follow this link for the full article as made available by the publisher.
Published December 17, 2018