Aging Detection Technology for Semiconductor Devices Combining Deep Learning and Evolutionary Algorithms
Cong Xu [Global ETS]
Wednesday, June 28th - 11:00 am
Abstract:
With the rapid development of technology, semiconductor devices play an increasingly important role in a wide range of fields. However, these devices age over time, leading to performance degradation, malfunctions and even system crashes. What's more, the recycling of aged chips as a major source of material for the counterfeit chip industry makes real-time aging detection of semiconductor devices critical. This paper introduces a technique for real-time aging detection of semiconductor devices utilizing the power of deep learning and evolutionary algorithms. Convolutional neural networks (CNNs) and recurrent neural networks (RNNs) are effectively used to evaluate and predict the aging status of devices. These identified features are then fed into evolutionary algorithm frameworks, such as genetic algorithms (GA) and particle swarm optimization (PSO), to identify and predict aging trends. The adaptive capability of the evolutionary algorithm ensures satisfactory generalization to various semiconductor devices. To illustrate this, we apply this approach to a commonly used semiconductor device. Through the analysis and prediction of real-time data, the aging trend of equipment is successfully identified, and timely maintenance and management are carried out. After extensive examination of experimental data, the proposed technique demonstrates higher accuracy and real-time performance compared to conventional aging detection methods. In addition, it provides real-time monitoring of its operation, providing valuable assistance to maintenance and management personnel. The research results help to improve the reliability and stability of semiconductor devices and lay a solid foundation for intelligent and automated maintenance.
Biography:
Cong Xu [Global ETS]
Xu Cong is currently a Ph.D. candidate at the University of South Florida, specializing in the field of digital signal processing in electrical engineering. His research mainly focuses on the application and challenges of hybrid artificial intelligence in chip testing, the current state of research on acoustic digital twin systems and their prospects in the field of autonomous driving, the 3D localization system of multiple sound sources based on TDOA and tetrahedral microphone array, and the research on the real-time evaluation method of semiconductor chip grade classification based on hybrid artificial intelligence technology. His research achievements have been applied in the fully automatic chip functional testing and universal tester research at Global ETS's automated detection production line, creating a lot of potential value.
Prior to this, he served as the founder of the International Hybrid Artificial Intelligence Association, a non-profit organization. He currently serves as a technical advisor for the GETS and USF research cooperation project. His professional skills include robot technology, artificial intelligence algorithms, and digital signal processing. He has won the first prize (third place) in the ROBOCUP China competition, a global robot competition.
Xu Cong has made significant contributions in the semiconductor industry, especially in the field of chip counterfeiting detection. His work and research in this field have promoted the development of the chip industry. This also includes his work at Global ETS, where his technology has been applied to the company's automated detection production line, bringing significant potential value.
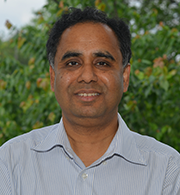
Dr. Diganta Das
For more information or questions regarding the technical program (including Professional Development Courses), contact the Conference Chair, Dr. Diganta Das.
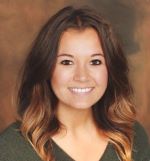
Karlie Severinson
For more information or questions regarding event logistics, exhibitions, and sponsorship, contact Karlie Severinson.
Top